Bias in AI: A Primer
(This article originally appeared on KDNuggets.com here. For more, visit https://www.kdnuggets.com/)
Those interested in studying AI bias, but who lack a starting point, would do well to check out this introductory set of slides and the accompanying talk on the subject from Google researcher Margaret Mitchell.
Bias in AI is as important now as it ever has been; it has always been an important topic, but it seems to be getting more attention as time goes on, attention it rightfully deserves. No longer an afterthought in relevant courseware and texts, AI bias and the related concepts of ethics, inclusion, and diversity are core and early topics in courses such as Stanford's CS224n: Natural Language Processing with Deep Learning, and the upcoming book from the Fast.ai folks titled Deep Learning for Coders with fastai and PyTorch, to name but two specific examples.
Aside from the gradually-increasing interest and inclusion of AI bias and ethics concerns from and by a wide variety of practitioners in their daily work, numerous researchers today are making a focused and very conscious impact as well. Margaret Mitchell is one such researcher working in this area. Mitchell (@mmitchell_ai) is a Senior Research Scientist in Google's Research & Machine Intelligence group. From her website, as pertains to what it is that she does:
My research generally involves vision-language and grounded language generation, focusing on how to evolve artificial intelligence towards positive goals. This includes research on helping computers to communicate based on what they can process, as well as projects to create assistive and clinical technology from the state of the art in AI.
While Margaret's work obviously goes well beyond the basics, she did give an introductory talk on the topic of AI bias for the winter 2019 iteration of Stanford's CS224n: Natural Language Processing with Deep Learning, the slides for which are available on the course website. The slides (and talk) are titled Bias in the Vision and Language of Artificial Intelligence, and are a great resource for those interested in AI bias and ethics but lack an entry point.
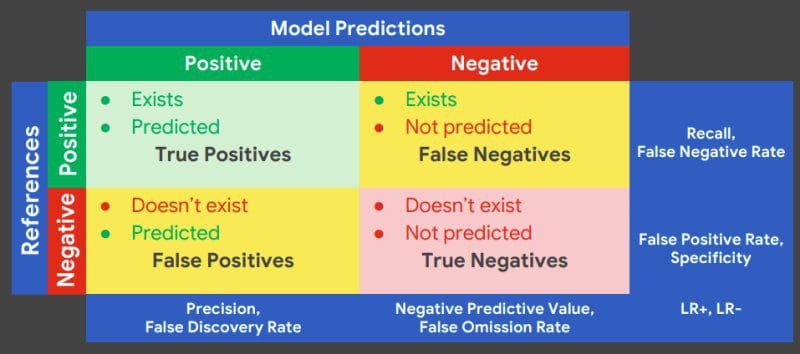
The talk is a self-contained, single class session of Natural Language Processing with Deep Learning (though it covers language, vision, and more "general" AI) and is easily digestible in one setting, either via the slides alone or alongside the accompanying talk video (see below).
First off, the slides cover (unsurprisingly) a number of specific biases and how they affect different aspects of AI systems, including human reporting biases, biases in data, interpretation biases, algorithmic biases, and many more. Also treated are a number of closely related concepts, such as the effects of prototype theory, fairness and inclusion, feedback loops, and unjust AI outcomes, to name a few. Additionally, questionable (and worse) projects such as predicting criminality from facial images are also discussed, along with their downfalls and biases in context.
Throughout, there is an emphasis on the human role in AI bias. Far from being an isolated, self-contained technology that exists in a vacuum separate from the humans that develop, train, curate data for, operate, and gain insights from, AI is a direct reflection of those humans which build and interact with these systems. As Mitchell states, "[i]t’s up to us to influence how AI evolves," and concrete examples are given of how we can do so.

Figure 2. Examples of human biases in data and human biases in data collection and annotation (from Margaret Mitchell's Bias in the Vision and Language of Artificial Intelligence slides).
As mentioned above, the content of the slides is also covered in a video of Mitchell's in-class talk which is also freely-available for anyone to view (see below), in which you can listen to the author expand upon the material in the slides in the hour long effort.
AI bias is a robust domain of study. More importantly, however, while it can be treated as its own entity worthy of devoted exploration, it is important that non-experts keep bias in mind in the context of AI in general. Simply put, bias in artificial intelligence is a concern for all, stakeholders and non-stakeholders, technical and non-technical, from researchers to engineers to practitioners to product designers and beyond.
It's up to everyone involved to do our collective best to build AI as free from bias as possible. As such, gaining a solid understanding of the relevant issues in AI bias is a must for everyone, and Margaret Mitchell's slides and accompanying talk are a great place to start.
Stay in the loop.
Subscribe to our newsletter for a weekly update on the latest podcast, news, events, and jobs postings.